Introduction to Micro LLMs: The Future of Efficient AI
In this post, I will explore the breakthrough of micro Large Language Models (LLMs) in artificial intelligence (AI). These models significantly reduce the parameter count—from hundreds of billions to as few as several million—while maintaining strong performance. This balance of efficiency and resource demands makes micro LLMs an increasingly attractive option for a wide range of applications. Recent innovations in reinforcement learning and efficient architecture design have further advanced the capabilities of these compact models.
What Are Micro LLMs?
Micro LLMs are essentially compact versions of traditional large language models. They are engineered to operate with far fewer parameters while still delivering robust performance on targeted applications. While conventional LLMs might use hundreds of billions or even trillions of parameters, micro LLMs typically range from a few million to a few billion parameters. This reduction in size makes them cost-effective to train and deploy and optimizes them for real-time applications on edge devices, mobile apps, and embedded systems.
Moreover, these models are being enhanced using modern techniques—such as reinforcement learning from human feedback and efficient attention mechanisms—that allow them to remain competitive despite their reduced scale.
Key Characteristics
- Efficiency: Micro LLMs require significantly less computational power and memory, making them suitable for deployment on devices with limited resources, including mobile phones and IoT devices.
- Customization: Their compact nature simplifies fine-tuning for niche tasks. This allows for the creation of domain-specific solutions in areas such as healthcare, finance, and customer support.
- Privacy: With lower data requirements and the potential for on-device processing, micro LLMs help enhance data privacy by reducing reliance on centralized servers.
- Speed: Optimized architectures lead to rapid inference, a critical factor for real-time applications, while also minimizing energy consumption.
- Scalability: The smaller size of these models enables their deployment across various platforms—from edge devices and smartphones to embedded systems—thus democratizing access to advanced AI capabilities.
Use Cases for Micro LLMs
Micro LLMs are finding applications across diverse industries. Here are a few examples:
- Customer Service Automation: These models can power chatbots that handle routine inquiries efficiently, enhancing customer satisfaction while reducing operational costs.
- Language Translation Services: They enable real-time translation, which is vital for bridging communication gaps in our globalized world.
- Sentiment Analysis: Micro LLMs can rapidly analyze text to gauge public opinion and customer sentiment, supporting marketing and brand strategy.
- Education: In adaptive learning environments, micro LLMs provide personalized tutoring and feedback, which is particularly beneficial for diverse learner needs.
- Predictive Maintenance: When deployed on edge devices in manufacturing, these models can monitor equipment and predict maintenance needs in real time.
- On-Device AI Applications: Recent advancements allow these models to run locally on smartphones and IoT devices, reducing latency and enhancing data security.
Advantages of Micro LLMs
- Accessibility: Lower resource requirements open up advanced AI capabilities to smaller organizations and individual developers.
- Cost-Effectiveness: Reduced training and inference costs make micro LLMs a budget-friendly alternative to larger models.
- Transparency and Explainability: With fewer parameters, these models are generally easier to interpret—an important factor in regulated industries.
- Domain-Specific Robustness: When trained on well-curated, domain-specific data, micro LLMs can deliver high levels of accuracy and reliability.
- Energy Efficiency: Their reduced computational load translates into lower operational costs and decreased energy consumption, promoting greener AI practices.
Challenges and Limitations
Despite the many benefits, micro LLMs have some limitations:
- Limited Capabilities: Their smaller scale may constrain performance on tasks requiring deep contextual understanding or abstract reasoning.
- Reduced Generalization: Micro LLMs might require extensive fine-tuning to adapt effectively to a variety of new tasks.
- Performance Constraints: In complex language tasks, they can underperform compared to larger models that possess a broader knowledge base.
- Customization Overhead: Tailoring these models for highly specialized applications may demand additional expertise and careful data preparation.
The Future of Micro LLMs
I believe that the future of micro LLMs is very promising. Ongoing research—such as improvements in mixture-of-experts, multi-head latent attention, and innovative reinforcement learning approaches—is continually enhancing their performance and efficiency. These developments will likely drive further improvements in customization, energy efficiency, and privacy, making advanced AI accessible on virtually every connected device. Moreover, global innovations, including those emerging from leading Chinese AI firms, indicate a strong shift toward low-cost, high-performance AI solutions.
Conclusion
In summary, micro LLMs are redefining the AI landscape by delivering efficient, cost-effective, and privacy-enhancing solutions ideal for resource-constrained environments. As research continues to refine their architectures and training methods, these models will democratize access to advanced AI capabilities and spur innovation across industries—from customer service and education to manufacturing and on-device applications. I am excited to see how micro LLMs will continue to evolve and transform our approach to deploying AI in the real world.
Interested in Implementing Micro LLMs?
Want to learn more about integrating micro LLMs into your applications? Explore our blog for more insights on AI optimization, or reach out to discuss how these efficient models can enhance your projects.
Related Articles
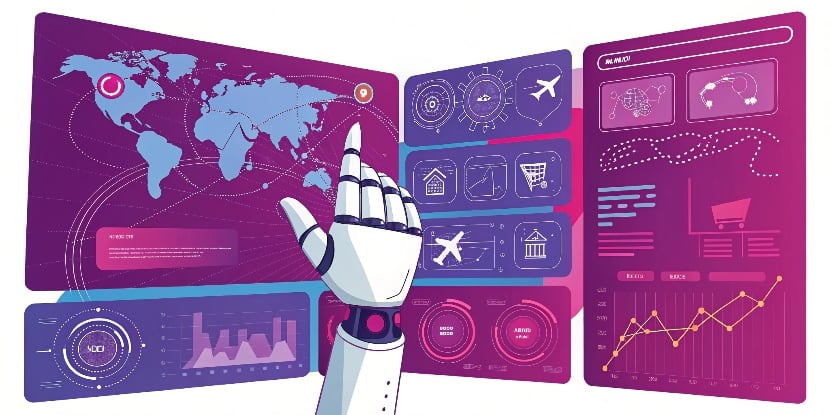
Manus AI: A New Frontier in Autonomous Intelligence
Explore Manus AI, one of the world's first fully autonomous AI agents. Learn about its capabilities, the technology behind it, and how it's redefining the way we interact with artificial intelligence.
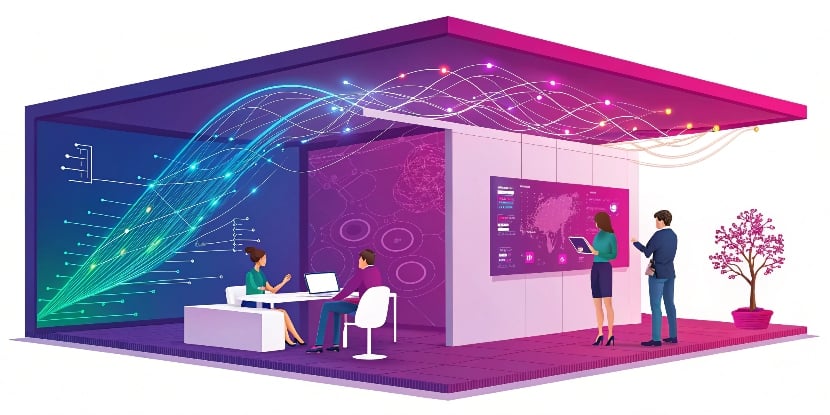
AI Integration Roadmap for Small Businesses
Discover the ultimate step-by-step AI integration roadmap for small businesses. Learn how to assess your needs, select the right tools, pilot projects, integrate AI into daily operations, and continuously optimize for growth and efficiency.